

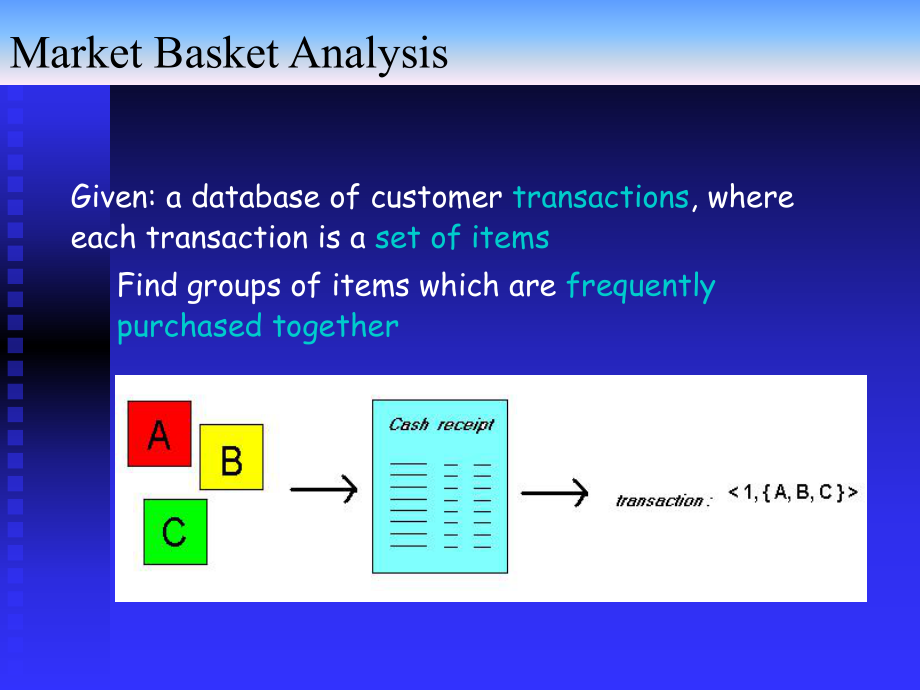


版權(quán)說明:本文檔由用戶提供并上傳,收益歸屬內(nèi)容提供方,若內(nèi)容存在侵權(quán),請進(jìn)行舉報或認(rèn)領(lǐng)
文檔簡介
1、 Customer buying habits by finding associations and correlations between the different items that customers place in their “shopping basket” Customer1Customer2Customer3Milk, eggs, sugar, breadMilk, eggs, cereal, bread Eggs, sugarMarket Basket Analysis (MBA)Given: a database of customer transactions,
2、 where each transaction is a set of itemsFind groups of items which are frequently purchased together Market Basket AnalysisMBA applicable whenever a customer purchases multiple things in proximity Goal of MBAAssociation RulesTransactions:Relational formatCompact formatItem: single element, Itemset:
3、 set of itemsSupport of an itemset I denoted by sup(I): card(I)Threshold for minimum support: Itemset I is Frequent if: sup(I) .Frequent Itemset represents set of items which arepositively correlatedBasic Concepts itemset sup(dairy) = 3 sup(fruit) = 3 sup(dairy, fruit) = 2 If = 3, then dairy and fru
4、it are frequent while dairy,fruit is not. Customer 1 Customer 2Frequent ItemsetsTransaction IDItems Bought1dairy,fruit2dairy,fruit, vegetable3dairy4fruit, cerealsq A,B - partition of a set of items q r = A B Support of r: sup(r) = sup(AB)Confidence of r: conf(r) = sup(AB)/sup(A)q Thresholds:u minimu
5、m support - su minimum confidence cr AS(s, c), if sup(r) s and conf(r) cAssociation Rules: AR(s,c)Transaction IDItems Bought2000A,B,C1000A,C4000A,D5000B,E,FFrequent Itemset SupportA75%B50%C50%A,C50%Min. support 2 50%Min. confidence - 50%Association Rules - ExampleThe Apriori algorithm Agrawalabcdc,
6、db, db, ca, da, ca, ba, b, db, c, da, c, da, b, ca,b,c,da,d is not frequent, so the 3-itemsets a,b,d, a,c,d and the 4-itemset a,b,c,d, are not generated.Apriori - ExampleAlgorithm Apriori: IllustrationuMining association rules is composed of two steps:TID Items1000 A, B, C2000 A, C3000 A, D4000 B, E
7、, F1. discover the large items, i.e., the sets of itemsets that have transaction support above a predetermined minimum support s.2. Use the large itemsets to generate the association rules A 3 B 2C 2A,C 2 Large support itemsMinSup = 2TID Items100 A, C, D200 B, C, E300 A, B, C, E400 B, E Database DA
8、B C D E Itemset CountA 2 B 3C 3E 3Itemset CountA, B A, C A, E B, C B, EC, E Itemset A,B A,C A,E B,C B,E C,E Itemset Count A, C 2 B, C 2 B, E 3C, E 2 Itemset Count B, C, E Itemset B, C, E 2 Itemset Count B, C, E 2 Itemset Count C1F1C2F2C2C3F3C3ScanDScanDScanDS = 22 3 3 1 3 1 2 1 2 3 2 Representative
9、Association RulesTransactions:A,B,C,D,EA,B,C,D,E,FA,B,C,D,E,H,IA,B,EB,C,D,E,H,IRepresentative Association RulesFind RR(2,80%)Representative Rules From (BCDEHI): H B,C,D,E,I I B,C,D,E,HFrom (ABCDE):A,C B,D,EA,D B,C,ETransactions:abcdeabcacdebcdebcbdecdeFrequent Pattern (FP) Growth StrategyMinimum Sup
10、port = 2Frequent Items:c 6b 5d 5e 5a 3Transactionsordered:cbdeacbacdeacbdecbbdecdeFP-treeFrequent Pattern (FP) Growth StrategyMining the FP-tree for frequent itemsets:Start from each item and construct a subdatabase of transactions (prefix paths) with that item listed at the end. Reorder the prefix
11、paths in support descending order. Build a conditional FP-tree.a 3 Prefix path:(c b d e a, 1)(c b a, 1)(c d e a, 1)Correct order:c 3b 2d 2e 2Frequent Pattern (FP) Growth Strategya 3 Prefix path:(c b d e a, 1)(c b a, 1)(c d e a, 1)Frequent Itemsets:(c a, 3)(c b a, 2)(c d a, 2)(c d e a, 2)(c e a, 2)Mu
12、ltidimensional ARAssociations between values of different attributes :RULES:nationality = French income = high 50%, 100%income = high nationality = French 50%, 75%age = 50 nationality = Italian 33%, 100%Multi-dimensional Single-dimensional Schema: Single-dimensional AR vs Multi-dimensionalQuantitati
13、ve AttributesProblem: too many distinct valuesSolution: transform quantitative attributes into categorical ones via discretization. Discretization of quantitative attributesConstraint-based ARApriori property revisitedMining Association Rules with ConstraintsMultilevel ARProductFam ilySectorDepartm
14、entF ro z e nR e frig e ra te dV e g e ta b leB a n a n a A p p le O ra n g e E tc .F ru itD a iryE tc .F re s hB a k e ryE tc .F o o d S tu ffHierarchy of conceptsFreshsupport = 20%Dairy support = 6%Fruit support = 1%Vegetable support = 7%q Support and Confidence of Multilevel Association RulesHier
15、archical attributes: age, salaryAssociation Rule: (age, young) (salary, 40k) ageyoung middle-aged old salarylow medium high 18 29 30 60 61 8010k40k 50k 60k 70k 80k100kCandidate Association Rules: (age, 18 ) (salary, 40k), (age, young) (salary, low), (age, 18 ) (salary, low)Mining Multilevel ARMining Multilevel ARMulti-level Assoc
溫馨提示
- 1. 本站所有資源如無特殊說明,都需要本地電腦安裝OFFICE2007和PDF閱讀器。圖紙軟件為CAD,CAXA,PROE,UG,SolidWorks等.壓縮文件請下載最新的WinRAR軟件解壓。
- 2. 本站的文檔不包含任何第三方提供的附件圖紙等,如果需要附件,請聯(lián)系上傳者。文件的所有權(quán)益歸上傳用戶所有。
- 3. 本站RAR壓縮包中若帶圖紙,網(wǎng)頁內(nèi)容里面會有圖紙預(yù)覽,若沒有圖紙預(yù)覽就沒有圖紙。
- 4. 未經(jīng)權(quán)益所有人同意不得將文件中的內(nèi)容挪作商業(yè)或盈利用途。
- 5. 人人文庫網(wǎng)僅提供信息存儲空間,僅對用戶上傳內(nèi)容的表現(xiàn)方式做保護(hù)處理,對用戶上傳分享的文檔內(nèi)容本身不做任何修改或編輯,并不能對任何下載內(nèi)容負(fù)責(zé)。
- 6. 下載文件中如有侵權(quán)或不適當(dāng)內(nèi)容,請與我們聯(lián)系,我們立即糾正。
- 7. 本站不保證下載資源的準(zhǔn)確性、安全性和完整性, 同時也不承擔(dān)用戶因使用這些下載資源對自己和他人造成任何形式的傷害或損失。
最新文檔
- 2025年度書畫藝術(shù)品市場代理銷售合同
- 2025年度建筑安全風(fēng)險評估與監(jiān)測委托合同
- 2025年度國際知識產(chǎn)權(quán)轉(zhuǎn)讓合同樣本
- 2024-2025學(xué)年福建省福州第十中學(xué)高一上學(xué)期期中考試歷史試卷
- 2025年公共租賃場地安全協(xié)議
- 2025年合作協(xié)議訂立與實(shí)施年
- 2025年醫(yī)院護(hù)師職責(zé)合同
- 2025年農(nóng)場土地租賃合同年協(xié)議書
- 2025年TBBS項(xiàng)目申請報告模稿
- 2025年焦化二甲苯項(xiàng)目規(guī)劃申請報告模范
- 酒店長包房租賃協(xié)議書范本
- 2 找春天 公開課一等獎創(chuàng)新教學(xué)設(shè)計
- 2025年江蘇護(hù)理職業(yè)學(xué)院高職單招語文2018-2024歷年參考題庫頻考點(diǎn)含答案解析
- 2025年江蘇南京水務(wù)集團(tuán)有限公司招聘筆試參考題庫含答案解析
- 【道法】開學(xué)第一課 課件-2024-2025學(xué)年統(tǒng)編版道德與法治七年級下冊
- 口腔門診分診流程
- 建筑工程施工安全管理課件
- 2025年春新外研版(三起)英語三年級下冊課件 Unit2第1課時Startup
- 2025年上半年畢節(jié)市威寧自治縣事業(yè)單位招考考試(443名)易考易錯模擬試題(共500題)試卷后附參考答案
- 處方點(diǎn)評知識培訓(xùn)
- 2025年新合同管理工作計劃
評論
0/150
提交評論